According to a report by Cognilytica on Data Engineering, Preparation, and Labeling for AI, the majority of time spent on AI projects, over 80%, is dedicated to data management tasks. These tasks include collecting and aggregating data, as well as cleaning and labeling it. As the utilization of data continues to expand, enabling artificial intelligence (AI) to push its capabilities further, this trend becomes even more prominent. As to be predicted, the global data labeling market is projected to reach a value of $13 billion by the year 2030, reflecting the growing significance of data management in AI development.
However, data annotation is not a simple task, and it's common to make mistakes along the way. In this blog post, we will explore the 5 most common data annotation mistakes and provide valuable tips on how to avoid them. By understanding these pitfalls, you can enhance the accuracy and reliability of your data annotations, leading to improved machine learning models.

Insufficient Guidelines or Instructions
One of the most significant mistakes in data annotation is providing insufficient or unclear guidelines to the annotators. Without clear instructions, annotators may interpret the labeling criteria differently, resulting in inconsistent annotations.
Tips to avoid this mistake
To avoid this, take the time to create detailed annotation guidelines. Clearly define the annotation task, provide examples, and address potential challenges. Regular communication and feedback with annotators are also essential to ensure a shared understanding of the annotation requirements.
Lack of Quality Control
Failure to implement robust quality control measures can severely impact the accuracy of annotated data. Without proper quality control, there is a higher chance of incorrect or inconsistent annotations slipping through.
Tips to avoid this mistake
To mitigate this, establish a quality control process that includes multiple rounds of annotation review, inter-annotator agreement checks, and regular feedback loops with annotators. Consider using annotation tools with built-in quality control features to streamline the process.
Not using the right tools
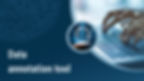
There are a number of different tools available for data annotation. Some tools are better suited for certain types of data or tasks than others. When annotators rely on inadequate tools, they encounter various challenges. Firstly, it leads to a manual and time-consuming annotation process, resulting in slower speeds and potential errors. Collaboration and version control become difficult, as annotators may work on different versions of data, leading to inconsistencies. Secondly, basic tools may lack the necessary annotation capabilities for complex datasets, limiting the potential of annotations. Scaling and managing large datasets also become challenging without appropriate features for bulk uploading, data management, and automated workflows.
Tips to avoid this mistake
To solve this problem, thorough research is essential to identify annotation tools that meet specific requirements. It is advisable to leverage AI-powered annotation platforms that automate parts of the process, reducing manual effort and improving accuracy. Furthermore, seeking integration with existing workflows saves time and ensures compatibility between annotation outputs and downstream processes.
Ignoring Bias and Subjectivity
Annotators bring their own biases and subjectivity into the annotation process, which can lead to skewed or inaccurate annotations. One consequence of this is the perpetuation of existing societal biases within the annotated data, leading to biased outcomes in machine learning algorithms. This can result in unfair treatment, discrimination, and inaccurate predictions. Additionally, subjective interpretations by annotators can introduce inconsistencies and variability in the annotations, reducing the reliability and reproducibility of the dataset.
Tips to avoid this mistake
This problem can be addressed by promoting awareness and providing guidelines on handling bias. Incorporate diverse perspectives and involve multiple annotators to reduce individual biases. Regularly evaluate and monitor annotations for potential bias and take corrective actions if necessary.
Failing to Iterate and Improve
Data annotation is an iterative process that requires continuous improvement. Many annotators make the mistake of assuming their initial annotations are perfect and final. However, as machine learning models evolve, annotation guidelines may need updates or adjustments.
Tips to avoid this mistake
To mitigate this issue, the annotators team should analyze the performance of the models trained on the annotated data and gather feedback from end-users. Incorporate this feedback into the annotation process, iterate on the guidelines, and provide ongoing training and support to the annotators. Regularly revisiting and improving the annotations will lead to higher-quality datasets and better-performing models.
Benefits of outsourcing to an experienced AI data annotation partner
Whether you’re trying to solve for speed, bias or any other type of challenge in the annotation process, working with an experienced and knowledgeable AI data solutions provider is one of the best ways to overcome obstacles. A data annotation partner can take the burden off of your organization and allow your machine learning teams to focus on developing cutting-edge technologies.
With an experienced team of data annotators and state-of-the-art data annotation tool suitable for various types of annotation, such as bounding boxes, 3D key points, segmentation, etc., Pixta AI is an excellent choice if you want to optimize costs in your data annotation process.
As a data annotation expert, we also provide free consultations if you have any issues with your in-house annotation team or your data annotation process.
Speak to one of our AI experts to learn how Pixta AI can help with your machine learning projects